ESG & Generative AI – Clash of the brave new worlds (Part 2 of 3)
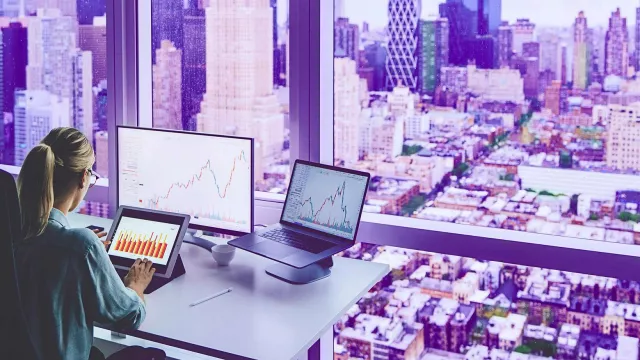
Part 2 in our thought leadership series exploring the opportunity for ESG and AI. This second article explores strategic decision making, plus uses cases of Gen AI in the sustainable finance space
The Empire Strikes Back, Toy Story 2, Terminator 2…… proof that the sequel is always better than the original, right? We certainly hope so, as here we are with part 2 in our mini-series on the collaborative possibilities of ESG and Generative AI in the sustainable finance space.
In this mini-series we are exploring how these new kids on the block are shaping the future and how, when combined, they have unlimited potential to drive the future of sustainable financing.
Following on from part 1, where we explored leveraging unstructured data, in this article we explore how Generative AI can help to provide key information for strategic decision making within your business in the context of sustainability.
We touch on the concept of ‘double materiality’, or a framework to evaluate sustainability from two perspectives: 1) Financial materiality (i.e. how sustainability issues impact a company’s financial performance; and 2) Impact materiality (i.e. how a company’s operations affect the environment and society).
It’s a key component of the European Union’s Corporate Sustainability Reporting Directive (CSRD), which, as noted in part 1 of our mini-series, is particularly relevant in Europe where double materiality needs to be considered under the CSRD rules; with approximately 50,000 businesses across a wide range of industries mandated to start reporting within these guidelines by 2025.
Strategic decision making
Let’s imagine your business sells a product that is currently made by a third-party supplier, and you have reached a position where you are considering bringing your production in-house. There are a number of considerations when weighing up this decision:
Financial – What is the investment required to bring the production in-house? What factors will impact the unit cost – labour, shipping, utilities etc? Do we have enough capital or will we need to fundraise?
Operational – Will the quality of the end product be the same? Is there an available market to hire the required skilled talent? Can we scale production quickly enough to meet our growing demand?
Sustainable – How is this going to impact our net zero ambitions? If we move production from country X to country Y, how will it impact the people working for our current supplier? How do we maintain compliance with multiple different jurisdictions’ rules?
Gathering the answers (and associated data) to all of these questions, in order to make a final decision relies on the double materiality model and broader sustainability concepts such as the triple bottom line (TBL), that is looking at three key areas: profit, people, and the planet.
To help us make similar decisions, Generative AI can be leveraged to obtain and analyse the hard data to provide detailed insights into the opportunity cost at hand.
Triple bottom line
Let’s take a look at some live examples of where triple bottom line is being put into practice in a variety of different markets:
Massterly – autonomous shipping
Massterly’s aim is to enable a shift in transport from road to sea; through cost-effective, safe and environmentally-friendly logistics.
Using their technology, ships receive a range of electronic data signals and the on-board computer uses advanced AI algorithms and machine learning to make safe decisions for navigation. This improves the climate impact of marine transport, whilst also significantly reducing costs and improving safety metrics: “75% of maritime accidents are caused by human error”.
Massterly delivered two autonomous vessels to leading Norwegian grocery distributor, ASKO, in September 2022, estimated to replace 150 daily truck journeys, eliminating 2 million kilometres of truck haulage, and saving 5,000 tons of CO2 per year.
In this context, AI provides multi-faceted benefits across the triple bottom line.
Coriolis – Automated ESG scoring
CoriolisESG enhances enterprise intelligence and resilience by measuring key entity data against globally-recognised frameworks, such as the UN Sustainable Development Goals and the EU Taxonomy.
Using geospatial analysis, the tool can generate a carbon emissions figure for a specific location with incredible accuracy. This is invaluable for businesses as it not only provides an actual carbon tonne figure for reporting, but also supports assessments and decision making where investment may be required to improve the results.
Leveraging this level of granular data in financial services is critical to ensuring transparency in reporting and analysis.
McKinsey – AI-driven scheduling
McKinsey is using AI to revolutionise traditional workforce management processes and provide dynamic, smart scheduling.
AI is being employed to dynamically optimize work schedules based on real-time labour market conditions.
“AI-driven solutions take significantly less time to schedule the workforce than current spreadsheet-based models, and the technology allows for a consistent and systematic approach, eliminating human bias and error, creating fairer planning schedules, and reducing the managerial bandwidth required to oversee the scheduling process.”
If we consider our earlier example, if you are managing your in-house goods production from afar, leveraging AI in this way is an effective means to mitigate the complexities of a new location, and ensures a more socially-equitable schedule for the local employees.
No such thing as a free lunch
All of these examples could lead you to believe that we should just down tools and let the computers do all the work for us. This, of course, isn’t reality, and human guidance is always going to be required – there is a reason that Microsoft have named their AI chatbot, CoPilot! There are many times where we need human instinct and contextualization to provide the bigger picture in decision making, particularly when it comes to the people impact of choices. It has been proven that companies with happy employees outperform in the long-run, and this is something that should be taken into consideration when making decisions fed by data and AI.
That being said, used correctly it can have material benefits in strategic decision making. In our production-dilemma example covered in this article, the business may previously have flown people around the world to assess the suitability of potential sites for their new production centre. They would then have needed to produce a comparative analysis of buy vs. build against their existing supplier, often with limited data points available.
In contrast, the ability of Generative AI to make thousands of calculations in a matter of seconds, and provide deep insights into the question at hand means that – used appropriately – our key decision makers don’t even need to get out of bed (and they certainly don’t have to turn their camera on for the next Teams / Zoom meeting!).
I’ll close with some considerations for the financial services market:
- How best can we incorporate the power of AI into our decision making processes?
- Which controls should we have in place to ensure that we apply appropriate context in our choices?
- How can we use this technology to assist our customers with their own ESG aspirations?
Stay tuned for part 3 as we explore these topics in the context of automating ESG reporting.