Synthetic data as a fintech solution towards digital justice and economic efficiency
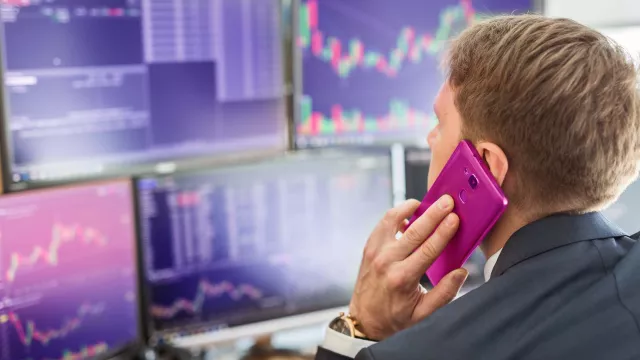
How do you extract the maximum value from data when there isn't enough to work with?
In this paper, Dr Bogachev and our other expert partners from the University of Leeds School of Mathematics explain how synthetic data can be generated to improve machine learning algorithms for financial applications, providing better forecasting and risk management. Potential applications could be improving the flow of funding to SMEs in emerging markets and modeling risk in unpredictable scenarios.
The phrase "data is the new oil" is often used to characterize the fundamental modern shift towards information-driven economy, seen by the World Economic Forum as the Fourth Industrial Revolution. This change is deepening and accelerating, pushed by trends such as ecommerce, the “Uberization” of services, open banking, and blockchain as well as factors such as environmental concerns and global economic slowdown. Currently, the global impact of COVID-19 has already enforced a rapid and massive transition to digital communication, remote working and online sales, putting many businesses at the brink of collapse.
In the "big data" era, advanced analytics, including machine learning (ML), serve as the backbone of the fintech industry by providing efficient computerized tools for data processing, most importantly due to their power to discover hidden relationships and patterns behind complex dynamic behaviors. However, ML is only as good as the data available to train and validate it. Advanced ML algorithms (e.g. deep/reinforcement learning) may require millions of data samples to be properly trained. While these are readily available in highly digitized spaces like web traffic or e-commerce, the financial domain suffers from with chronic data scarcity. This is caused by a lack of historical data, obsolescence as economic regimes shift, data bias/quality, and privacy constraints. These issues affect smaller/regional institutions and their customer bases in particular. Forecasting potentially disastrous future events (black swans) is of the utmost importance for risk management, but as these are naturally rare they are under-represented in historical data.
The challenge of chronic data shortage has been increasingly noted, but the response remains immature. One solution is an aggregator/data broker service, whereby data supplied by institutions are anonymized and pooled together. In addition to multiple legal and commercial hurdles, a potential pitfall here is that aggregation of different risks may mask complex dependencies and increase bias. Nevertheless, this may be a viable option for the regulator, provided they possess adequate ML expertise and computational power. An example is the. “smart cube” reporting model in Austria. Regulators would also need to be prepared to outsource some of their capabilities to the fintech sector, which should therefore stay "bid ready".
A deeper incentive for centralized data collection, processing and assessment relates to risk management. Institutions are currently required, for example under IRRBB, to reconstruct the entire probability distribution behind their assets and then calculate risk metrics such as Value-at-Risk or Expected-Shortfall to ensure compliance. But data scarcity means the accuracy of reconstruction may be poor and of little value in reported benchmarks. Compliance merely creates the illusion of security if it is built on poor data. We argue that risk margins must be modelled directly in the extreme value domain, for example using a Peaks-over-Threshold (POT) approach and generalized Pareto distribution (replacing the usual normal distribution). But again, the scarcity of observed “outliers” presents a problem.
An innovative alternative is to use the ML statistical toolkit and generate synthetic data that emulates real-life samples or the perceived “environment”. The initial goal is to learn the latent probabilistic model behind the complex behavior via Bayesian predictive inference based on sequential updates from prior knowledge and available data. The posterior distribution can then be used to generate multiple data samples, representing any desired region in the distribution space. Note that difficulties caused by analytic intractability and/or computation complexity of calculating the posterior, can be resolved using Markov Chain Monte Carlo (MCMC) algorithms. In collaboration with Finastra, we have recently piloted this approach in the simple use case of loan prepayments via combining the Cox survival model with POT techniques to generate unlimited synthetic datasets, enabling, for example, extensive stress testing including different behavioral patterns.
We believe that the synthetic data approach is set to become instrumental in enhancing the efficiency and fidelity of ML algorithms, which can be trained against a huge variety of dummy scenarios and thus become better prepared for unseen extreme events. This is crucial in achieving greater resilience and democratization of digital resources, which is important for a speedy recovery from COVID-19 (especially for SMEs and regional banks) and for fostering future sustainable growth.
The University of Leeds School of Mathematics works with Finastra to co-develop an innovative data synthetic generator vital piece of analytics that will make relevant advanced AI/ML models. This project has already been integrated into the financial inclusion Finastra CSR project called “The Trust Machine”.
The Trust Machine program aims to reduce the SME funding in developing markets and create jobs that will help these countries to build a sustainable economy. The first step is to create a bank of data that will feed the predictive and optimization models.
This is an example of how an open-platform approach to financial software introduces focused expertise through industry/academic partnerships, to the benefit of the industry and society.
Written in collaboration with Georgios Aivaliotis, János Gyarmati-Szabó (University of Leeds), Nitin Iyer, Arnaud Picut and Mariusz Podsiadło (Finastra).