AI: Unlocking the opportunities in corporate banking
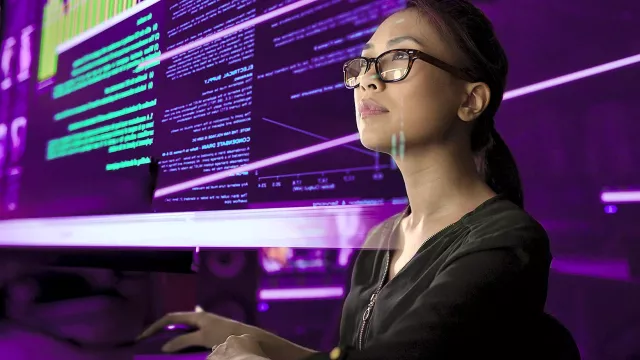
It’s becoming increasingly clear that artificial intelligence (AI) – and the plethora of next-generation technologies associated with it – is not only here to stay, but plays a fundamental role in the transformation of corporate banking.
Although the financial sector was one of the first industries to deploy AI and machine learning on a large scale, because of the pace of change, there is still lots to learn in terms of how to maximize its potential, while also navigating the risks.
At Finastra’s recent Europe Corporate Banking Conference, industry experts discussed some of the key opportunities for AI across corporate banking, and explored ways in which financial institutions (FIs) can truly unlock the full power of data through AI.
Practical applications
From enabling operational efficiencies to enhancing the client experience, AI offers tremendous opportunities for innovation within corporate banking. Two of the use cases that came to light during the conference relate to back office and frontline efficiency.
Streamlining the back office
“The focus is increasingly shifting from robotic process automation (RPA) towards the ways in which AI can help with decision making,” suggests Marc Smith, CEO of Conpend. “This is fundamentally transforming document processing and enabling straight-through processing. For example, imagine a world in which you submit a loan application, it’s approved, and you receive the money – all within five minutes. This is being made possible with AI. In fact, everything that is workflow-driven can potentially be replaced with a large language model.”
Dermot Canavan, COO, Trade and Trade Commodity Finance at ING, explains how AI is driving efficiencies in ING’s compliance checking processes: “Compliance checking has traditionally been an extremely paper- and people-heavy, tedious process, with lots of room for error. AI and large language models are enabling a much more automated compliance checking process, resulting in better data with fewer mistakes and gaps.”
Revolutionizing frontline effectiveness
Enrico Muti, partner at McKinsey & Company, suggests that AI – and generative AI, specifically – could fundamentally change how corporate banks engage with clients. “Gen AI can semi-automate pitch and proposal preparation, mine data to suggest client-specific actions, summarize insights from client interactions, and draft client emails,” he explains. “These technologies are also being used to vastly improve the ways in which chatbots can support clients with queries, acting as virtual assistants to help them navigate the bank offerings.”
McKinsey & Company’s partner Markus Röhrig adds: “By automatically interpreting and completing technical documentation, gen AI offers many opportunities to improve key processes like time consuming onboarding.”
AI is also increasingly being used by software developers to increase operational efficiencies and improve the client experience. A bank’s developers can use AI to help them write code, write unit tests, and auto-document their code.
Maximizing the power of AI
So, what are the fundamentals that FIs need to get right to implement and scale AI successfully?
Marc suggests the first step is to truly understand what AI technology can do. “Once you appreciate the capabilities, you can start to imagine what’s possible,” says Marc. “Once you’ve defined what it is that you want AI to do, it’s about thinking how it can be rolled out across the organization, and how to make the most of the skills, capabilities and data that already exist within the organization.
Speaking to Russ Rawlings, Regional VP, Financial Services and Public Sector Leader UK&I, Databricks post-event, he revealed that in order to execute a data and AI strategy effectively, it’s important to focus on three key areas: people, process, and platform.
1. People
It’s important to consider the entire organization when designing and implementing an AI strategy, says Russ: “This requires having a clear understanding of the roles and needs of all the different users of AI – including data scientists, data analysts and data engineers, as well as business users. You also need key people at every level to help drive change and ensure that AI is being embraced across the business.”
As demonstrated by the use cases outlined above, although AI can help by automating lots of manual processes and assisting with decision making – it still needs people to be truly effective. “The human element is always going to be key. For example, an AI system can extract data and correct it where it made mistakes, but you still need human oversight to ensure that process is robust – and to be able to step in at the appropriate points if it’s not going so well,” Dermot Canavan explains.
“To get everyone on board with the shift to AI, it’s imperative to communicate your plans, seek alignment on methodology, and build a strong community and culture where best practice and success stories are recognized and celebrated. Most importantly, it’s about being agile and flexible so that everyone can respond to changes effectively,” explains Russ.
2. Process
As with any new technology, adopting AI and large language models requires a sound plan with clearly defined processes.
Russ says: “Implementing an AI and data strategy involves significant change, and planning the course of your change is essential. Big process change starts with defining the principles and rules; going back to those helps to make decisions and to move things along.”
Lewis Liu, Chief AI Officer at Sirion, emphasizes the importance of implementing a robust risk management process: “This should include a framework to test AI models and clear rules to support validation. Human oversight and intervention are also crucial, particularly in terms of facilitating end-to-end data processing.”
“Of course, an AI and data strategy can’t exist on its own and needs to align with business strategy. Effective governance is also key to executing a successful strategy. Although complex, good governance is fundamental to all stages of the process,” explains Russ.
“To develop a clear governance framework for AI – and its associated data – an FI needs to understand what it’s doing, record what it’s done and have the evidence to support its actions. Good governance is about more than just ensuring you meet the necessary data privacy rules – it’s also about responsible AI, having high quality data, and ensuring the ethical use of AI,” says Dermot.
3. Platform
The third essential element of a good data and AI strategy is finding the right platform. Businesses across all industries are increasingly moving towards platforms based on ‘data lakehouse’ architecture – an open, unified foundation for both unstructured and structured data – with around three quarters of global enterprises having adopted this model, according to Russ.
The date lakehouse concept blends the benefits of data lakes and data warehouses, allowing FIs to better scale AI. Russ explains: “When built on an open foundation, a data lakehouse can easily integrate with the entire AI ecosystem to enable machine learning, business intelligence, and predictive analytics. What’s more, when combined with generative AI, this model provides a powerful data intelligence platform that can democratize data and AI across the entire organization.”
Building momentum
Many industry commentators refer to AI – and GenAI in particular – as the fourth industrial revolution. Russ says: “It’s democratizing data for users across the world. FIs can’t fall behind, and must think carefully about how they embrace the revolution.”
Marc sums it up: “Because of the amount of data they hold and the processes they manage for the economy, banks have a massive opportunity to move the needle. The future of our industry could look very different. So even though implementing change at this scale isn’t easy, the best thing to do is just to start – just do it.”